We propose a time varying 3D (4D) analysis scheme which will rely on a: (i) spatial-temporal dependency framework so as to decrease computational cost and make the analysis suitable for various engineering applications, (ii) predictive modelling so that we can fill out missing elements of an object geometry which may not be visible from the sensors due, for instance, to occlusions, (iii) 4D computer vision with semantically enriched information to make the results searchable and retrievable and simultaneously reduce processing time and (iv) incorporation of non-visible data (such as hyper-spectral and thermal imaging) to identify other properties such as material decay phenomena. |
Spatio-temporal dependency means that a 3D model at a current time instance is generated taking into account information of the same object on previous reconstructions (temporal dependency) and common surface properties (spatial dependency). Predictive refers to the ability to select regions of interest to be reconstructed differently by fusing/integrating selective 3D capturing with respect to surface material and geometric properties. Semantic enrichment allows 4D models to be enriched with high-level textual/visual semantics so as to: (i) assist documentation through object linking and (ii) perform 3D digitalization under a cost-effective (joint reconstruction). |
Our Objectives
Objective 1: Novel cost-effective 4D (3D geometry plus time) computer vision models and tools |
We develop 4D computer vision models that will represent, apart from the 3D objects’ geometry, their evolution through time. The proposed 4D modelling goes beyond a simple aggregation of independent time-varying 3D reconstructions since this results in an arduous, time consuming process. 4DBeyond exploits information from previous 3D reconstructions and surface objects of similar properties to accelerate reconstruction time, while simultaneously keeping the resolution efficiency the same. |
Objective 2: 4D modeling of non-visible data such as from hyper-spectral & thermal sensing |
4DBeyond implements novel algorithms, not yet extensively studied in the literature, on 4D reconstruction of data from non-visible sources such as hyper-spectral & thermal sensing. These types of data describe with high accuracy the materials of the objects. The goal is to automatically create detailed geometric maps depicting in 3D space the positioning of these phenomena and thus stimulating preventive actions to avoid the decay expansion. Research challenges refer to the use of new descriptors (or modification of the current RGB ones) to identify regions of interest within the non-visible streams invariant from the Field of View (FoV) and the lighting conditions and to affine geometric transformations. Image matching algorithms and outliers’ removal using the RANSAC (Random Sample Consensus) procedures will be investigated. |
Objective 3: Semantically enrichment of the 4D models to make them retrievable and searchable |
The derived models are semantically enriched to make them retrievable and searchable using historic, cultural, functional or constructional information. Semantics also decrease the computational cost for the 4D modelling since the details of the reconstruction are tuned to the “significance” of a surface in terms of history values, effect of previous restorations, etc. |
Objective 4: 4D Modelling in tangible/intangible Cultural Heritage (CH) |
We apply the 4D computer vision research in the analysis of tangible and intangible CH data. Regarding tangible CH, we provide to CH community a time varying model through which (i) they can estimate the effect of different conversation materials and techniques for CH object preservation, (ii) the impact of environmental and natural factors that affect objects’ stability (e.g., humidity, temperature, etc.) and the evolution of their weak parts, (iii) they can better document the monument by understanding how it behaves against natural hazards, (iii) they can relate different assets with respect on how they behave in time. |
Objective 5: Detecting human behaviors across a network of multiple visible/non-visible cameras |
We analyze the behavior of humans who are monitored across a network of cameras (either overlapped or disjoint) using RGB visible sensors or hyper-spectral & thermal imaging. The goal is to localize the position of humans in the 3D space and time and then using novel machine learning tools such as deep learning to extract information regarding the activities they execute. This is important for several applications such as evacuation, safety and security or monitoring of public infrastructures. |
Objective 6: 4D Modelling in inspecting road/tunnel infrastructures |
One of the greatest challenges facing transport operators and engineers is the fast and efficient inspection, assessment, and safe operation of existing infrastructures such as tunnels and of RI in general. The goal of this research is to provide a computational framework for inspecting a RI against cracks, defects and other anomalies. 3D information in time is exploited to show the degree of importance of a defect and its expansion and time evolution in the civil infrastructures. |
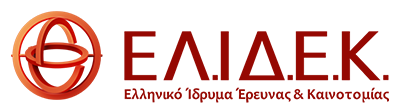
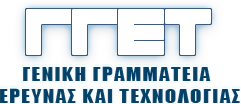
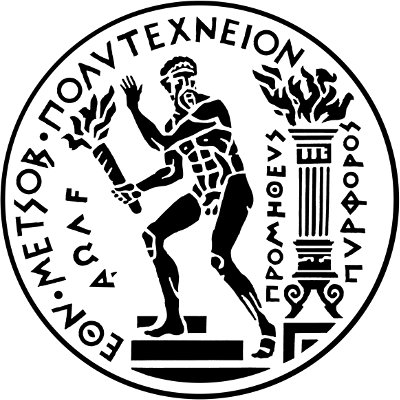